January 7, 2025
Data Integration in Healthcare
Healthcare is a sector where timely and accurate data can make the difference between life and death.
Digital transformation in healthcare is revolutionizing the industry, driving significant improvements in patient care, operational efficiency, and innovation.
At the heart of this transformation lies data—spanning electronic health records (EHRs), medical devices, genomics, and patient-generated health information.
Integrating these diverse data sources is essential for creating a unified, actionable view of patient health, enabling personalized care, streamlined workflows, and informed decision-making.
In this article, Dirox delves into the critical role of data integration in fueling healthtech, exploring its potential to make healthcare smarter, more efficient, and more connected.
I. The Importance of Data Integration in Healthcare
Data integration involves the seamless combination of information from various sources to provide a unified view of patient health. It plays a pivotal role in bringing together data from EHRs, medical devices, genomics, and other systems to enhance care delivery and operational efficiency.
Key Benefits
Enhancing Patient Care: Integration creates a 360-degree view of patients, enabling more accurate diagnoses and treatment plans.
Streamlining Workflows: By automating data sharing, healthcare organizations can reduce inefficiencies and administrative burdens. For example, in the context of improving operational efficiency, Verisys, the US-based leading provider of health care credentialing services, leverages automated tools to gather and verify data from over 2,500 sources multiple times daily.
Data-Driven Decisions: Integrated data supports preventive care initiatives and personalized treatments by leveraging analytics and AI insights. For instance, predictive analytics tools at Kaiser Permanente have lowered hospital re-admission rates by 12%.
Emerging Use Cases
Real-Time Responses in Health Crises: During emergencies like COVID-19, integrated systems enable real-time monitoring and resource allocation. For example, integrated health data networks were pivotal in tracking infections and allocating resources efficiently.
Fraud Detection and Resource Optimization: Advanced analytics identify anomalies and optimize the use of limited healthcare resources. The National Health Service (NHS) in the UK adopted fraud detection systems integrated with healthcare data and have successfully prevented, detected, and recovered £400 million of fraud.
II. Challenges in Healthcare Data Integration
Data Silos and Interoperability Issues
Healthtech systems often struggle to connect legacy systems with modern platforms, leading to fragmented data silos that hinder integration.
For example, a HIMSS survey found that 57% of physicians face interoperability challenges in maximizing healthtech. This lack of interoperability can lead to delayed care, redundant testing, and medical errors, ultimately compromising patient outcomes.
Data Privacy and Security
Data integration relies heavily on digital data exchange; therefore, ensuring the security of patient information is critical. The average cost of a healthcare data breach, according to IBM’s 2022 report, is $10.1 million.
Balancing the need for data integration with compliance requirements, such as HIPAA and GDPR, presents a significant challenge in safeguarding sensitive patient information.
Lack of Standardization
Healthcare data is generated by a variety of sources, including electronic health records (EHRs), medical devices, and patient self-reports. This diversity in data formats and inconsistent quality across systems makes integration complex and time-consuming.
Without standardized data formats, definitions, and protocols, comparing and integrating data from different sources can be difficult.
For example, one group of clinicians might define a cohort of asthmatic patients differently than another group. Similarly, if you ask two clinicians what criteria are necessary to identify someone as diabetic, you might receive three different answers. There may simply be a lack of consensus regarding specific treatments or cohort definitions.
Scalability for Small and Mid-Sized Organizations
Budgetary and infrastructure limitations often impede smaller organizations from adopting robust data integration solutions.
Cloud-based solutions like AWS and Microsoft Azure are emerging as cost-effective alternatives for such organizations.
III. Traditional vs. Emerging Technologies in Data Integration
Current Technologies
ETL (Extract, Transform, Load)
This foundational technology is widely used for consolidating data from different sources into a centralized database. ETL systems work well for static data and batch processing but face challenges in handling real-time data.
For example, hospitals often use ETL to integrate data from radiology systems into central EHRs for diagnostic purposes.
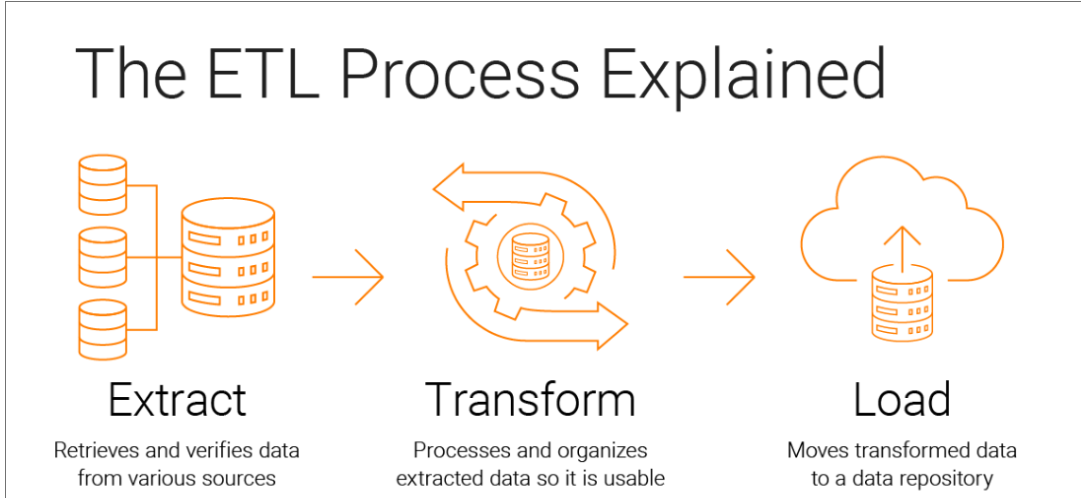
EDR (Enterprise Data Replication)
EDR provides real-time replication of data across systems to ensure consistency. It’s particularly valuable in large, distributed healthcare networks, allowing seamless updates between branches.
An example is the use of EDR in regional hospital networks to synchronize patient records for emergency care.
FHIR (Fast Healthcare Interoperability Resources) Standards
FHIR enables different healthcare systems to communicate by defining standard data formats and elements.
Many organizations have successfully implemented FHIR to connect over 50 disparate systems, improving collaboration and reducing manual data entry errors.
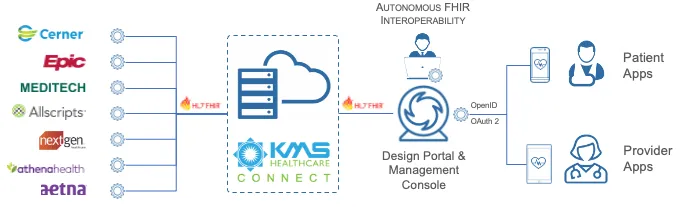
A compelling example is LDPath, a UK-based surgical pathology service developed by Dirox that integrates with the National Health Service (NHS) to streamline histopathology workflows. This integration supports the efficient exchange of information between hospitals and LDPath, covering tasks such as user creation, case management, diagnosis, and monitoring.
By leveraging modern web frameworks and secure networks, LDPath exemplifies how traditional integration technologies combined with FHIR standards can enhance operational efficiency and improve patient outcomes.
Emerging Innovations
AI and Predictive Analytics
Artificial intelligence is revolutionizing data integration by enabling real-time insights and predictive modeling.
AI predictive analytics is one of the most major trends in healthtech right now. It examines massive amounts of patient data derived from genetic, environmental, and lifestyle factors to identify those at risk of diabetes or heart disease.
By identifying patterns and connections in this data, AI systems can generate prediction models that are more accurate than traditional ways for predicting patient outcomes. Predictive analytics powered by AI could save the US healthcare system $150 billion each year by 2026.
Blockchain
Regarding security, blockchain technology enhances healthcare data security and privacy by creating decentralized, encrypted health records that are less vulnerable to breaches than traditional systems.
In pharmaceutical supply chains, blockchain provides transparency and traceability, addressing issues like counterfeit drugs and inefficiencies.
Blockchain’s immutable record-keeping and real-time tracking capabilities are transforming both healthcare data management and pharmaceutical logistics.
IoT (Internet of Things) Integration
IoT devices like wearables and smart medical equipment provide continuous health monitoring and real-time data transmission. For example, continuous glucose monitors used by diabetic patients send real-time data to healthcare providers, enabling proactive management of blood sugar levels.
Wearables and IoT devices, such as Apple Watch and Dexcom’s CGM system, are transforming healthcare by enabling continuous health monitoring and proactive management of conditions. These devices now offer advanced features like glucose monitoring, sleep tracking, and atrial fibrillation detection, empowering patients to take charge of their health.
IoT integration allows devices like connected glucometers and blood pressure monitors to transmit real-time data to healthcare providers for personalized treatment plans.
Natural Language Processing (NLP)
Natural Language Processing (NLP) is revolutionizing healthcare by converting unstructured data from electronic health records (EHRs) into actionable insights, thereby enhancing patient care. By analyzing clinical notes, research articles, and patient feedback, NLP enables healthcare providers to make informed decisions, leading to improved outcomes.
Additionally, NLP streamlines administrative tasks such as documentation and coding, reducing the burden on healthcare professionals and allowing them to focus more on patient care.
The integration of NLP into healthcare systems is a significant advancement towards more efficient and effective patient care.
Advanced Platforms and Tools
Cerner and Epic Systems
These leading EHR platforms are integrating emerging technologies like AI and IoT, creating hybrid systems that enhance data visualization and predictive analytics. Epic’s integration with wearable devices, for instance, provides clinicians with comprehensive insights into patient activity levels and recovery progress.

Cloud-Based Platforms
Cloud solutions like AWS HealthLake and Microsoft Azure Health Data Services offer scalable environments for storing and analyzing integrated healthcare data. These platforms support real-time analytics and provide tools for advanced data modeling, making them ideal for organizations of all sizes.
IV. Best Practices for Successful Data Integration
Data Strategy and Governance
Define clear governance policies to ensure data quality and regulatory compliance. Prominent frameworks like COBIT (Control Objectives for Information and Related Technologies) and DAMA-DMBOK (Data Management Body of Knowledge) provide structured approaches for data governance, emphasizing principles like accountability, data quality, and risk management.
For example, at Johns Hopkins, by adopting robust governance frameworks, they can establish accountability for data ownership and align integration efforts with organizational goals.
Organizations should prioritize a unified strategy that ensures consistent data usage across departments. For example, a unified data dictionary needs to be established to ensure all stakeholders use consistent terminology and understand data elements in the same way. It should define what constitutes a “patient visit” or “admission” across the entire organization.
Understanding Your Data
Conduct comprehensive profiling and assessment of data sources to address format inconsistencies and quality issues.
For example, UCLA Health uses data profiling initiative to identify anomalies, ensuring that only high-quality data entered their integrated systems.
Common file formats encountered in healthcare data include:
- HL7 (Health Level 7): A widely used standard for exchanging clinical information between systems.
- FHIR (Fast Healthcare Interoperability Resources): A modern standard for API-based data sharing, enabling faster and more flexible integration.
- CSV (Comma-Separated Values): Often used for exporting and importing tabular data but prone to formatting issues.
- XML (Extensible Markup Language): Used for structured data exchange, though bulkier than newer formats like JSON.
- JSON (JavaScript Object Notation): Lightweight and ideal for modern APIs, increasingly adopted in healthcare for real-time integration.
Among these, FHIR is emerging as a preferred standard due to its flexibility, compatibility with APIs, and ability to support real-time data exchange, making it a cornerstone of modern healthcare interoperability efforts.
Focusing on data lineage—tracking the origin, transformations, and destination of data—can further enhance accuracy and trust. This is particularly important for reconciling data from disparate systems, such as aligning lab results with patient records in EHRs.
A strong focus on data lineage—tracking the origin and transformation of data—can further improve accuracy.
Leveraging Cloud-Native Architectures
Adopt platforms like AWS, Microsoft Azure, or Google Cloud for scalable and cost-efficient data processing.
These cloud platforms provide robust, flexible tools for managing vast and complex healthcare datasets, supporting features such as high availability, real-time analytics, and seamless integration with various healthcare applications. They enable healthcare organizations to process data from multiple sources while ensuring that the infrastructure scales according to demand.
For example, the Deep Data Research Computing Center (DDRCC) at Stanford University uses AWS for precision medicine research, which involves integrating and analyzing large-scale multimodal data. Thanks to that, DDRCC has improved the scalability, security, and elasticity of its health management systems.
By embracing cloud-native architectures, healthcare organizations can unlock the full potential of their data, drive innovation, and provide better, more efficient care to patients worldwide.
Interoperability Standards
For seamless data communication, interoperability standards must be adopted. The three main groups of health interoperability standards are:
Messaging (or data exchange) standards like HL7 and DICOM. They define protocols for exchanging data between healthcare systems. They enable systems to communicate patient information, such as lab results, diagnostic images, or appointment details, in a standardized format.
Terminology standards like SNOMED CT, ICD-10 (CIE-10), and LOINC. These standards ensure consistent definitions and coding of medical terms, conditions, and procedures, enabling clear communication across different systems and providers.
Document standards like CDA, CCR, and CCD. They define how health records are structured and shared, ensuring that comprehensive and readable patient information can be exchanged between providers and systems.
Robust Security Measures
Implement multi-layered security frameworks, including encryption, two-factor authentication, and real-time threat detection. Regular audits and penetration testing can identify vulnerabilities early.
Attackers frequently target vulnerabilities to gain access to EHRs, which house sensitive information such as personal data, medical histories, and insurance details. Every year, healthcare data breaches impact millions, resulting in billions of dollars in damages and eroding public trust.
To mitigate these risks, implementing advanced encryption techniques and conducting regular security drills are essential steps to prevent breaches while ensuring secure data integration.
End-User Experience Design
Design user-friendly dashboards and interfaces tailored to the needs of clinicians, administrators and patients.
For example, in a clinical setting, clinicians often work under time constraints and high pressure. Interfaces should be simple, with large, easily readable fonts, clear icons, and concise information. Dashboards should prioritize the most critical patient information, such as lab results or medication schedules, to enable fast decision-making. Features like voice command capabilities or touchscreen optimization are also helpful for users who may be wearing gloves or have limited mobility.
Administrators, on the other hand, might need more complex data sets for operational purposes. Layouts should focus on organizing large volumes of data in a digestible format, using tools like interactive charts, filters, and customizable reports. Color contrast is especially important in healthcare settings, where users may have varying degrees of visual ability. High contrast between text and background (e.g., black text on a white background) ensures readability for users with low vision.
For patients, interfaces should be designed with ease of access in mind, including clear fonts, intuitive navigation, and options for language preferences. For instance, a telemedicine app might feature large buttons and a straightforward flow for scheduling appointments, ensuring that elderly patients or those with limited tech experience can easily navigate the system.
In healthcare UX/UI design, ensuring accessibility across these various needs not only enhances the user experience but also contributes to improved outcomes and greater efficiency in care delivery.
Continuous Training and Support
Invest in ongoing training programs to ensure staff are adept at using integrated systems.
Regular refresher courses and role-based training sessions can help staff keep up with updates and new features that may be introduced to the systems. For example, clinical staff may require specific training on how to use a new decision support tool integrated into their EHR, while administrative staff might need guidance on using new reporting features.
Tailored support models are also crucial to ensure smooth system usage and address user concerns promptly. Help desks and support teams should be easily accessible, offering assistance via phone, email, or live chat to resolve any technical issues or questions that may arise. A dedicated IT support team familiar with the healthcare environment can troubleshoot problems quickly, ensuring that critical patient data or workflows are not disrupted.
Beyond training, it's important to create a feedback loop where staff can voice concerns, share suggestions, and report difficulties with integrated systems. This feedback can inform ongoing support strategies and allow organizations to fine-tune their training programs to meet evolving needs.
Conclusion
Healthcare data integration is transforming the industry by enhancing patient care, improving operational efficiency, and enabling innovative solutions. While challenges such as interoperability and data security remain, adopting emerging technologies and adhering to best practices can unlock the full potential of a connected healthcare ecosystem.
By embracing integration, healthcare organizations can move toward a more patient-focused future.
Get in touch with Dirox to explore how data integration can take your business to the next level!
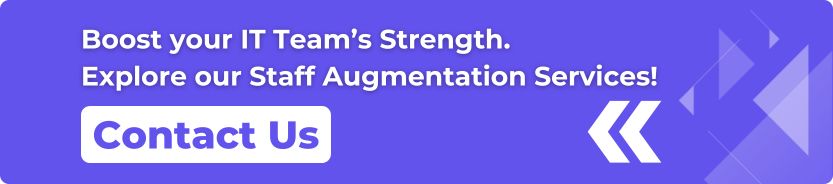