March 12, 2025
Top 10 Programming Languages for AI You Need to Know
In 2025, the global AI market is projected to reach $243.72 billion. AI-driven technologies like recommendation systems, fraud detection, and natural language processing have become essential in our daily lives, making AI expertise more valuable than ever.
At the heart of AI’s advancements lies programming. The choice of programming language significantly impacts the efficiency, scalability, and performance of AI models.
Whether you're developing a deep learning system or a simple AI chatbot, selecting the right language is crucial for success.
This article explores the top 10 programming languages for AI, highlighting their strengths, weaknesses, and ideal use cases.
How AI and Traditional Coding Differ?
The Core Difference: Data vs. Instructions
Traditional programming and AI development take fundamentally different approaches to problem-solving.
Traditional coding relies on explicitly defined rules and instructions, where a developer writes precise algorithms to process input and generate output.
In contrast, AI is data-driven, meaning it learns patterns and makes decisions based on large datasets without requiring explicit programming for every possible scenario.
For example, a rule-based spam filter in traditional coding might flag emails containing specific keywords (e.g., "free money" or "limited offer"). An AI-based spam filter, however, would analyze thousands of emails, learning patterns in spam messages and refining its accuracy over time.
The table below highlights key differences:
Key Concepts in AI
Understanding the core AI concepts helps clarify why AI programming differs from traditional coding:
Machine Learning (ML): Algorithms that learn from data to make predictions or decisions without being explicitly programmed for every case. Examples include recommendation systems like those used by Netflix and Amazon.
Deep Learning (DL): A subset of ML that utilizes neural networks with multiple layers, making it highly effective for image recognition, speech processing, and autonomous driving. Training deep learning models requires high computational power and large datasets.
Natural Language Processing (NLP): AI’s ability to understand, interpret, and generate human language. Applications include virtual assistants like Siri and Google Assistant, as well as automated chatbots and translation services.
Computer Vision: AI’s capability to interpret and analyze visual information from images and videos. It powers facial recognition, autonomous vehicles, and medical imaging diagnostics.
Emphasis on Libraries and Frameworks
AI development often relies on powerful pre-built libraries and frameworks that simplify complex computations:
TensorFlow and PyTorch for deep learning
scikit-learn for machine learning algorithms
OpenCV for computer vision tasks
NLTK and spaCy for NLP applications These tools enable developers to focus on model training and optimization rather than writing algorithms from scratch.
Iteration and Experimentation
Unlike traditional programming, AI development is highly iterative. It involves:
Data collection and preprocessing
Model training and fine-tuning
Testing, validation, and performance evaluation
Continuous improvement with more data and refined parameters
For example, AI-based fraud detection systems continuously improve by analyzing new transaction data, while traditional rule-based systems would require manual updates to adapt to new fraud techniques.
Top 10 Programming Languages for AI
Python
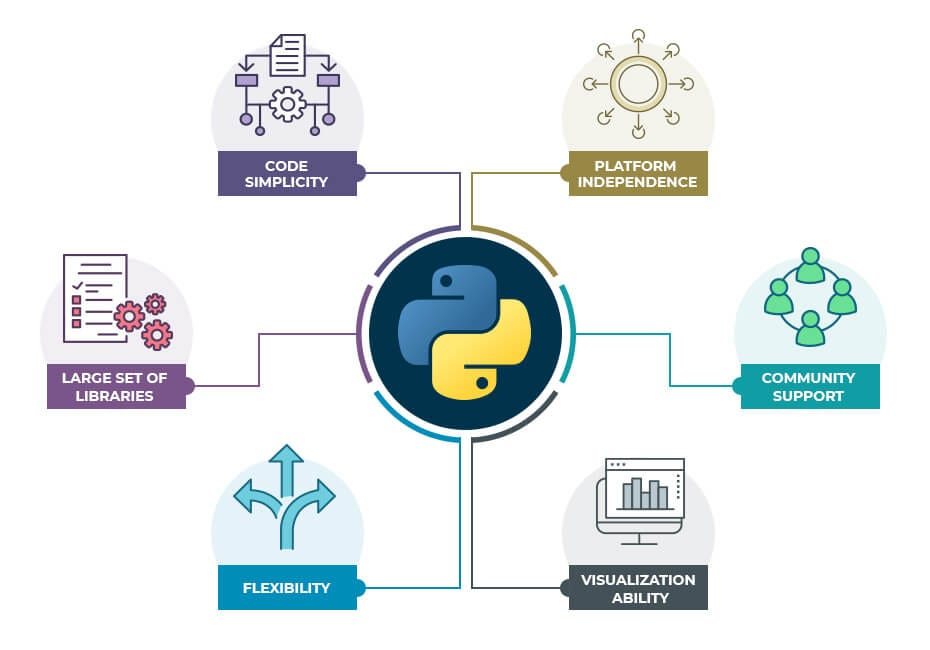
Python is the most widely used programming language for AI and machine learning, with a 70% adoption rate among AI developers. Its user-friendly syntax, extensive libraries, and adaptability make it the go-to choice for AI projects across industries.
Strengths of Python in AI
Simplicity and Readability: Python’s intuitive syntax makes it easy for beginners and experienced developers alike to build AI models efficiently.
Extensive Libraries and Frameworks: Python provides a rich ecosystem of libraries, including:
- NumPy for numerical computing
- scikit-learn for machine learning algorithms
- TensorFlow and PyTorch for deep learning
- NLTK and spaCy for natural language processing
Versatility: Python is used in various AI applications, such as:
- Neural networks and deep learning models
- Image recognition and computer vision (OpenCV)
- Predictive analytics and recommendation systems (Netflix, Amazon)
- Chatbots and NLP-based automation (Siri, Google Assistant)
Large Community and Open-Source Support: With thousands of contributors worldwide, Python offers vast resources, making troubleshooting and learning easier.
Weaknesses of Python in AI
Slower Performance: As an interpreted language, Python runs slower than compiled languages like C++ or Java, which may affect performance in large-scale AI applications.
Limited Native Mobile Development: Unlike Java (Android) or Swift (iOS), Python lacks robust frameworks for mobile AI applications.
High Computational Demands: AI models in Python often require GPUs and cloud-based solutions to handle large datasets efficiently.
Python stands as the dominant language for AI development, offering powerful tools and libraries that facilitate machine learning, deep learning, and data-driven innovations.
R
R is a free, open-source programming language designed for statistical computing and data visualization.
R’s strength lies in its ability to process and analyze complex datasets, making it a valuable tool in AI research and data-driven applications.
Strengths of R in AI
Excels in Statistical Computing: R is optimized for statistical modeling, probability analysis, and hypothesis testing, making it ideal for AI applications that rely on structured data.
Rich Ecosystem of Packages: R has an extensive collection of libraries that enhance AI development:
- Tidyverse for data manipulation
- Caret for machine learning workflows
- ggplot2 for advanced data visualization
- TensorFlow for R to integrate with deep learning frameworks
Data Exploration and Visualization: With powerful visualization capabilities, R helps data scientists interpret AI model outputs and identify key insights.
Strong Community Support: A large, active community continuously develops new libraries and solutions, expanding R’s capabilities in AI and statistical analysis.
Weaknesses of R in AI
Slower Performance for Large-Scale Applications: R is not as fast as Python or Java when handling massive datasets or high-performance AI tasks.
Limited Flexibility for Broader AI Tasks: While R excels in statistical modeling, it is less suitable for deep learning, natural language processing, and production-scale AI deployments.
Steeper Learning Curve: R’s syntax and statistical focus can be challenging for those without a background in data science.
R remains a top choice for AI applications that require statistical modeling, data mining, and visualization.
However, its limitations in flexibility and performance make it more suitable for early-stage AI research and experimentation rather than large-scale AI deployments.
Java
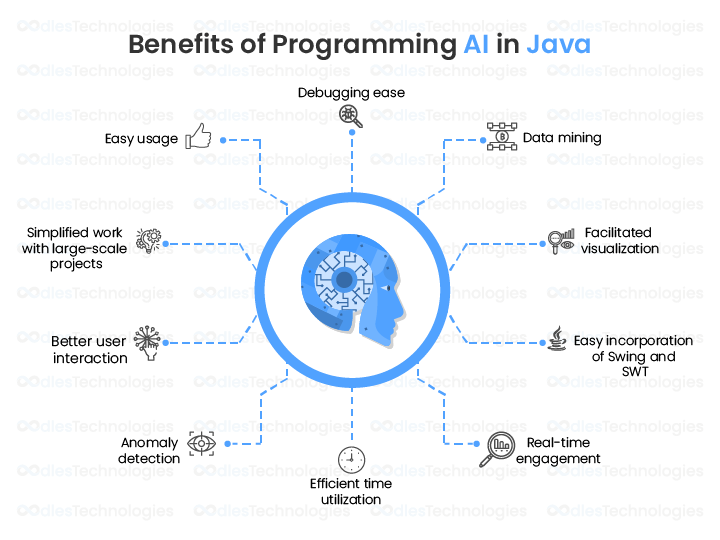
Java is a widely used, general-purpose programming language known for its portability, scalability, and reliability, making it an excellent choice for enterprise-level AI applications.
With its platform independence—allowing AI applications to run on any system with a Java Virtual Machine (JVM)—Java remains a powerful option for AI deployment in business environments.
Strengths of Java in AI
Portability and Scalability: Java’s write once, run anywhere (WORA) capability ensures AI applications can be deployed across different systems without modification.
Platform Independence: Java AI applications can operate seamlessly on multiple operating systems via the JVM.
Automatic Memory Management: The garbage collection feature enhances performance and reduces memory leaks in AI models.
Strong Support for Multithreading: Java’s ability to execute multiple tasks simultaneously makes it efficient for AI applications that require parallel processing.
Extensive Ecosystem: Java provides a rich selection of AI-related libraries and frameworks, including:
- DeepLearning4j – Supports deep learning and neural networks on the JVM.
- Weka – A machine learning library for classification, regression, and clustering.
- Mallet – Provides tools for natural language processing (NLP) and topic modeling.
Weaknesses of Java in AI
More Verbose Code: Compared to Python, Java requires more lines of code to implement the same AI functionalities, increasing development time.
Slower for Computation-Heavy Tasks: While Java is faster than Python, it is not as optimized as C++ for high-performance AI applications that require intensive computations.
Java remains a solid choice for enterprise AI applications, offering stability, scalability, and cross-platform support.
While it may not be as lightweight as Python or as fast as C++ for computation-heavy tasks, its strong ecosystem and platform independence make it an excellent option for AI-driven business solutions.
C++
C++ is a powerful, high-performance programming language essential for AI applications requiring low-level hardware control, real-time processing, and high efficiency.
It is widely used in AI systems that demand millisecond latency and scalability, such as high-frequency trading, robotics, and embedded systems.
Strengths of C++ in AI
High Performance and Efficiency: As a compiled language, C++ enables fast execution with minimal memory usage, making it ideal for large-scale AI computations.
Low-Level Hardware Control: C++ allows direct memory manipulation and hardware acceleration, optimizing AI model execution on Intel, AMD, and ARM chips.
Parallel Computing and Multithreading: C++ supports advanced concurrent processing, crucial for AI tasks that require real-time data processing and complex computations.
Standalone Executable Programs: C++ AI applications can run without dependencies across multiple platforms, ensuring reliable and scalable deployments.
Weaknesses of C++ in AI
Steeper Learning Curve: C++ has a complex syntax and requires manual memory management, making it harder to learn compared to languages like Python.
Less AI-Specific Library Support: While C++ supports AI frameworks (e.g., TensorFlow, Caffe2, OpenCV, ONNX), most machine learning tools are primarily optimized for Python.
By integrating C++ with Python, developers can balance research-friendly prototyping with high-performance production deployment.
Julia
Julia is an emerging programming language gaining traction in the AI community due to its suitability for AI and machine learning applications.
Introduced in 2012, it combines the ease of use of languages like Python with the speed of low-level languages like C++.
Strengths of Julia in AI
High Performance: Julia’s Just-In-Time (JIT) compilation allows it to process vast amounts of data efficiently, outperforming Python in computationally heavy AI tasks.
Rapid Prototyping: Julia enables quick iteration of AI models while maintaining high execution speed, making it ideal for research and development.
Interoperability: Julia seamlessly integrates with other languages like Python, R, and C, allowing AI developers to leverage existing libraries and frameworks.
Built-in Parallelism: Julia natively supports multi-threading and distributed computing, essential for training complex AI models on large datasets.
Weaknesses of Julia in AI
Smaller Ecosystem: Compared to Python, Julia has fewer AI-specific libraries and a smaller developer community, leading to limited support.
Compatibility Issues: Frequent updates to the Julia language can sometimes cause backward compatibility challenges, affecting long-term AI projects.
Julia is an exciting alternative to Python for AI development, offering high-speed performance and powerful numerical computing capabilities for AI researchers and developers working on performance-critical applications.
JavaScript
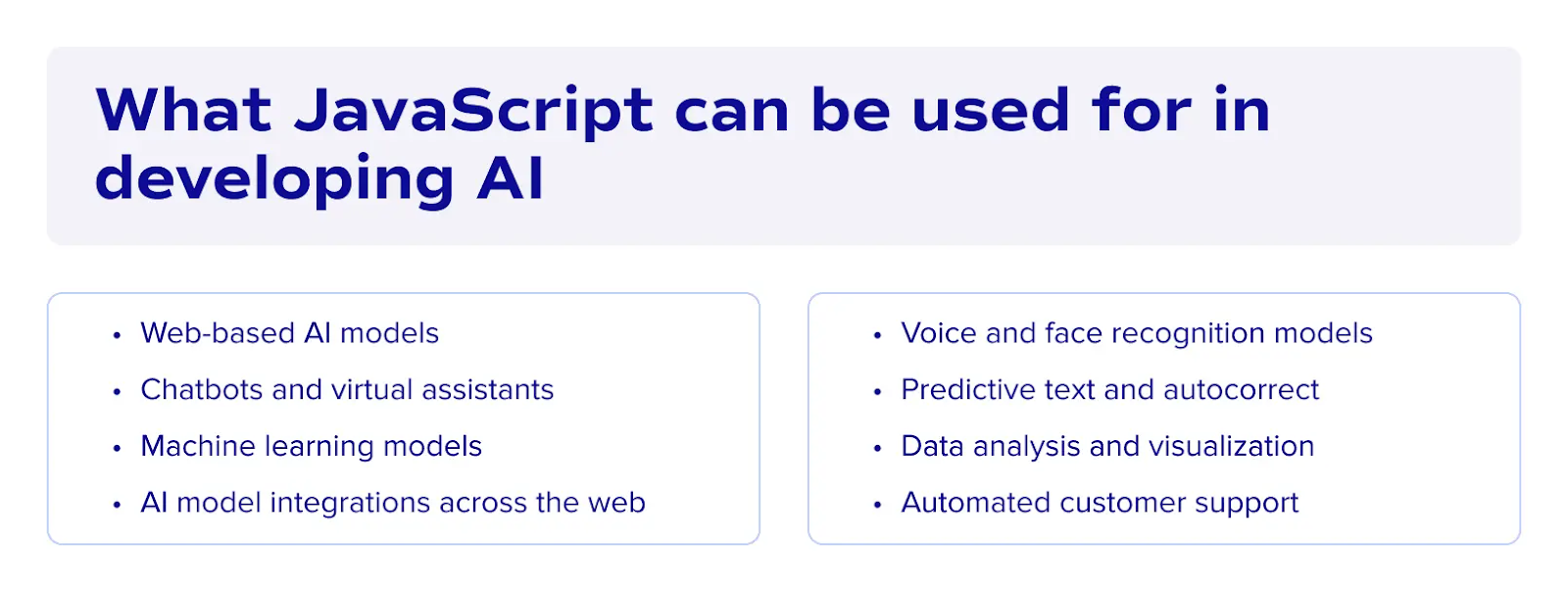
JavaScript, traditionally known for web development, is carving out a niche in AI, particularly for web-based AI applications.
With over 98.3% of all websites using JavaScript as of 2024, its ubiquity makes it an appealing choice for integrating AI functionalities into interactive applications.
Strengths of JavaScript in AI
Real-Time AI Applications: JavaScript enables dynamic AI-powered applications such as chatbots, virtual assistants, and recommendation engines.
TensorFlow.js for Browser-Based Machine Learning: This framework allows developers to train and deploy models without server dependencies. Companies like Google leverage JavaScript’s AI potential, using TensorFlow.js in projects such as Teachable Machine, which enables users to train AI models directly in a web browser.
Cross-Platform AI Solutions: JavaScript’s compatibility across operating systems and devices help AI applications function seamlessly across platforms. With 62% of global website traffic now originating from mobile devices, JavaScript’s role in mobile-friendly AI applications is becoming more crucial.
Weaknesses of JavaScript in AI
Computational Constraints: JavaScript struggles with large-scale computations and deep learning models while Python and C++ remain superior in heavy AI tasks.
Security Concerns: AI applications running in browsers are susceptible to security threats, such as data leaks and unauthorized model access.
As AI adoption continues to rise, JavaScript’s role in AI is set to expand, particularly in web-based and mobile-friendly AI applications.
With advancements in WebAssembly and WebGL, JavaScript’s performance for AI-related tasks is improving, making it a more viable option for lightweight machine learning models.
Lisp
Lisp, one of the oldest high-level programming languages, has played a significant role in AI development.
Initially created in 1958 by John McCarthy, Lisp was specifically designed for symbolic computation, making it a natural fit for early AI research.
However, in recent years, Lisp has lost traction as ML and neural networks—heavily reliant on statistical approaches—have dominated the AI landscape.
Strengths of Lisp in AI
Adaptability: Lisp’s dynamic nature allows for quick modifications and prototyping in AI applications.
Symbolic Expression Processing: Ideal for knowledge representation, automated reasoning, and symbolic AI.
Homoiconicity: Code and data share the same structure, enabling powerful metaprogramming and AI logic construction.
Used in Pioneering AI Systems: Played a crucial role in AI tools like DART, Macsyma, and early NLP systems.
Weaknesses of Lisp in AI
Declining Popularity: Lisp ranks outside the top 20 languages in the TIOBE Index and is used by less than 1% of developers.
Limited in Modern AI: Lacks robust libraries and frameworks for machine learning and deep learning.
Weaker Numerical Performance: Not optimized for large-scale numerical computations compared to Python, C++, or Julia.
Industry Shift to Statistical AI: The rise of data-driven AI models has made statistical programming languages more relevant.
Lisp’s legacy in AI is undeniable—it was the backbone of early AI research and pioneered many concepts still relevant today. However, the shift towards statistical learning and neural networks has significantly reduced its mainstream adoption.
Its role in the future of AI will likely remain specialized rather than widespread.
Prolog
Prolog (short for PROgramming in LOGic) is a high-level programming language widely recognized for its strength in logic programming and symbolic reasoning.
Developed in the early 1970s, Prolog has found applications in areas such as expert systems, natural language processing (NLP), and knowledge representation.
Unlike procedural languages, Prolog follows a declarative paradigm, where programmers define a set of facts and rules, and the Prolog interpreter infers conclusions automatically.

Strengths of Prolog in AI
Logic Programming & Symbolic Reasoning: Prolog is ideal for AI applications that require rule-based reasoning, making it well-suited for expert systems, theorem proving, and automated planning.
Declarative Nature: Unlike imperative languages, Prolog focuses on what needs to be solved, rather than how to solve it, allowing for concise knowledge representation and efficient problem solving.
Pattern Matching & Recursion: The language efficiently processes recursive structures, making it useful for parsing languages, decision trees, and complex queries in AI systems.
Weaknesses of Prolog in AI
Performance Limitations: Prolog struggles with large datasets and intensive numerical computations, making it less suitable for modern big data-driven AI.
Machine Learning Capabilities: Unlike Python, Prolog lacks deep learning and statistical AI frameworks, reducing its relevance in modern AI research.
Versatility: While powerful for symbolic AI, Prolog is not as adaptable as Python or C++ for general-purpose AI development, such as computer vision and reinforcement learning.
Although Prolog is not a mainstream AI language like Python or Java, it remains valuable in symbolic AI applications such as expert Systems used in medical diagnosis, legal reasoning, and automated decision-making.
Despite its limitations in modern data-driven AI, Prolog’s logical reasoning capabilities ensure it remains relevant in niche AI domains.
Haskell
Haskell is a purely functional programming language that offers a unique approach to software development, making it an interesting candidate for AI applications.
Haskell offers lazy evaluation and a strong type system yet very limited AI-specific libraries which have slowed its adoption in mainstream AI development.
Strengths of Haskell in AI
Efficient Data Processing: Haskell’s functional programming paradigm enables seamless manipulation of complex data structures.
Computational Efficiency: Unlike imperative languages, Haskell employs lazy evaluation, meaning computations are only executed when needed. This can lead to significant performance improvements in AI applications by optimizing resource usage.
Robust Type System for AI Safety: Haskell’s strong and static type system helps prevent a wide range of programming errors at compile time, reducing the chances of runtime failures.
Weaknesses of Haskell in AI
Difficult to Learn for Beginners: Haskell’s abstract syntax and functional paradigm can be challenging for developers accustomed to imperative languages like Python or Java.
Limited AI Libraries and Frameworks: Haskell’s ecosystem is not rich, making it harder for Haskell developers to find extensive pre-built tools for AI experimentation and deployment.
Haskell provides a solid foundation for developing AI applications where correctness is non-negotiable.
Haskell may not replace Python as the dominant AI language, but this emphasis on correctness and efficiency ensures it will continue to play a vital role in high-assurance AI systems.
Scala
Originally developed in 2004 by Martin Odersky, Scala has gained popularity in industries that require high-performance computing.
Companies like Twitter, LinkedIn, and Netflix use Scala to build AI-powered applications that process vast amounts of data in real-time.
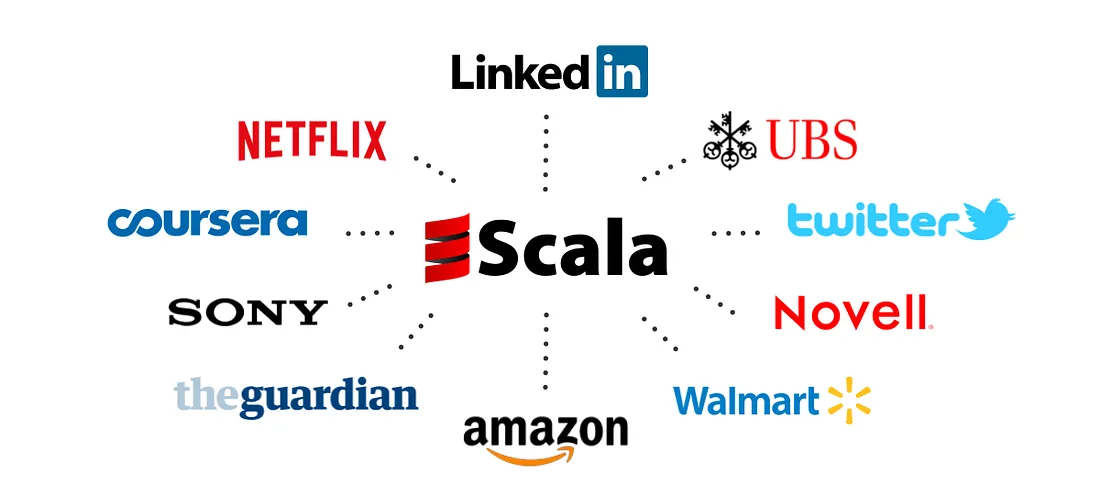
Strengths of Scala for AI
Functional & Object-Oriented Hybrid: Combines functional programming (immutability, higher-order functions) with object-oriented principles, offering flexibility in AI model design.
Multiprocessing & Parallel Computing: Supports distributed computing, making it ideal for handling large-scale AI and machine learning tasks. For example, Scala is used in Apache Spark, a widely adopted big data framework that enables AI-powered data processing across clusters.
Interoperability with Java: Runs on the Java Virtual Machine (JVM) and seamlessly integrates with Java libraries, providing access to a vast AI and machine learning ecosystem.
Challenges of Using Scala for AI
Steep Learning Curve: Functional programming concepts like immutability, monads, and higher-order functions can be difficult for beginners.
Slower Compile Times: Compared to Java, Scala's compile times can be up to 10 times slower due to complex type inference and advanced language features.
Scala remains a strong choice for developers working with big data and AI at scale.
While it may not be as beginner-friendly as Python, its scalability and parallel computing capabilities make it a valuable asset in AI-driven applications.

IV. What Factors Determine the Appropriate AI Programming Language?
From project requirements to team expertise, each element influences the efficiency, scalability, and success of AI implementation.
Below are key factors to consider when determining the most appropriate AI programming language:
1. Project Requirements
The nature of your AI project significantly impacts language selection. Consider the following:
Type of AI Task: Different AI tasks benefit from different languages:
- Machine Learning (ML): Python (TensorFlow, Scikit-learn)
- Deep Learning (DL): Python, Julia (PyTorch, Flux.jl)
- Natural Language Processing (NLP): Python (NLTK, SpaCy), Java (Stanford NLP)
- Computer Vision (CV): Python (OpenCV), C++ (fast processing)
Data Volume and Complexity:
- Large-scale AI applications require languages with efficient memory management and scalability (e.g., C++, Scala).
Performance Requirements:
- Real-time AI systems (e.g., autonomous vehicles) require languages with low latency and high-speed execution (e.g., C++, Rust).
Hardware Resources:
- GPU support is essential for deep learning; Python and Julia integrate well with CUDA and TensorFlow.
- Memory constraints impact the feasibility of high-level languages in edge computing.
2. Existing Infrastructure
If the AI system must integrate with an existing tech stack, language choice may be dictated by compatibility.
Enterprise applications: Java, C#
Big data platforms: Scala (Apache Spark)
3. Team Skills and Expertise
Choose a language that aligns with your team's expertise.
Python is widely adopted due to its ease of learning and abundant AI resources.
Functional languages like Haskell or Scala require specialized knowledge.
4. Available Libraries and Frameworks
Strong AI ecosystems improve development speed.
Python: TensorFlow, PyTorch, Keras, Scikit-learn
Java: Deeplearning4j
C++: OpenCV, TensorRT for performance-critical applications
5. Community Support and Resources
A language with a large developer community offers better support, documentation, and troubleshooting resources: Python and R have extensive AI and data science communities.
6. Development Time and Cost
Consider both learning curve and development speed:
Python has shorter development cycles due to its simplicity.
C++ and Java may take longer but offer performance advantages.
7. Deployment Environment
Where the AI model will be deployed matters:
Cloud-based AI: Python, Java (AWS, GCP, Azure)
Edge AI and IoT devices: C++, Rust for performance and security
8. Scalability
If the project will scale, consider languages that support distributed computing:
Python + Apache Spark for big data AI applications
Scala for large-scale data processing
V. What skills complement AI programming expertise?
Several skills can complement AI programming expertise. These skills enhance a programmer's ability to develop, deploy, and manage AI solutions effectively.
1. Technical Skills

Mathematics and Statistical Analysis
AI models rely heavily on linear algebra, probability, and statistics to process and analyze data.
Understanding concepts like regressions, derivatives, and matrix operations is essential for machine learning (ML) and deep learning (DL).
Data Manipulation & Data Structures
Efficient handling of structured and unstructured data is key to AI development.
Skills in data preprocessing, feature engineering, and dataset management improve model accuracy and performance.
Programming Package & Library Proficiency
AI frameworks such as TensorFlow, PyTorch, Scikit-learn, OpenCV, and NLTK enable faster AI model development.
Setting up and managing environments with virtual environments, Docker, and cloud platforms optimizes workflow.
Software Development Principles
Building scalable AI solutions requires expertise in software architecture, version control (Git), and API development.
Familiarity with microservices, containerization (Docker, Kubernetes), and cloud deployment enhances efficiency.
Understanding of Hardware
AI performance depends on efficient hardware utilization, including GPUs, TPUs, and edge computing.
Knowledge of parallel computing, memory optimization, and model quantization can lead to faster, more efficient AI systems.
2. Soft Skills
Problem-Solving and Critical Thinking: AI development involves constant debugging, optimization, and fine-tuning models to solve complex problems.
Creativity and Innovation: Developing new AI-driven solutions requires out-of-the-box thinking, especially in NLP, computer vision, and automation.
Effective Communication: AI professionals must explain models and insights to non-technical stakeholders, making clear communication essential.
Adaptability: AI evolves rapidly—staying updated with new techniques, tools, and research papers ensures long-term expertise.
Ethical Judgment: AI impacts society in profound ways; understanding bias, fairness, and responsible AI development is critical.
Collaboration and Knowledge Sharing: AI is a collaborative field—engaging with open-source projects, research communities, and developer forums fosters innovation.
3. Practical Experience
Project Work
Applying AI knowledge through real-world projects, Kaggle competitions, and GitHub contributions helps build credibility.
Writing blogs, publishing tutorials, and showcasing projects demonstrate expertise to potential employers.
Continuous Learning
Taking AI-specific online courses, certifications, and attending workshops keeps skills sharp.
Engaging with AI conferences, research papers, and industry trends ensures long-term growth.

Conclusion
Selecting the right programming language is crucial for AI development, impacting efficiency, scalability, and project success.
We explored how AI programming differs from traditional coding, examined the strengths and weaknesses of top languages, and outlined key selection factors like performance needs, team expertise, and scalability.
AI is constantly evolving, with new tools and frameworks emerging. Continuous learning is essential to stay ahead. The best way to start is by experimenting with a language that fits your goals and leveraging online resources and hands-on projects.